Introduction
Enriched real-world data studies combine primary data collected directly from physicians and patients with existing (secondary) data such as electronic medical records (EMRs), insurance claims or established registries. This approach takes advantage of the strengths of both primary and secondary sources, yielding maximum scientific benefit while allowing sponsors to answer more questions within a single study. By utilizing readily available data, enriched studies can focus data collection efforts on difficult-to-capture data, thus enabling more efficient research with less burden on care providers and patients. This article discusses strategies to leverage the increasing availability of linkable clinical, administrative and patient-reported data for more powerful approaches to safety, effectiveness and health outcomes research. We explore design and implementation considerations and include a case study in the hectic, high-patient-volume clinical setting of type 2 diabetes primary care.
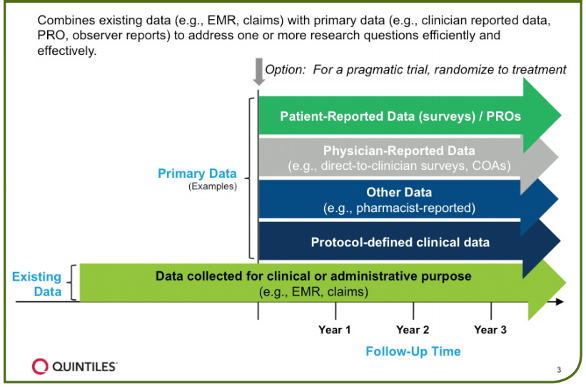
Figure 1. Features of enriched real-world data studies
Overview of Enriched Studies
The concept behind enriched studies is that existing data, such as EMRs and administrative claims, are leveraged to quickly and efficiently construct a patient cohort and acquire baseline data. Targeted primary data is then collected prospectively only for important data elements not reliably collected in existing data (Figure 1)
Significant value can then be added by coupling existing data with critical data elements that can only be obtained directly from physicians and patients, such as patient reported outcomes (PROs)1 and other Clinical Outcome Assessments2 which provide additional insights not available through existing data sources. For this reason, enriched studies are particularly useful in studying endpoints like preferential prescribing of one treatment over another, reasons for treatment switches or discontinuation, treatment adherence, healthcare resource utilization, and longitudinal PROs including Quality of Life (QoL). Randomization can be introduced to this combination of data sources for a pragmatic trial.
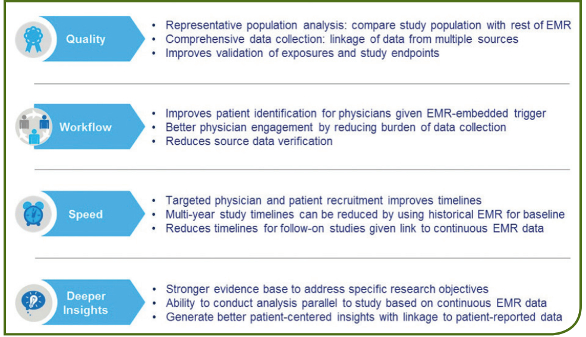
Figure 2. Benefits of enriched real-world studies
Advantages of Enriched Studies
In addition to deepening research insights, enriched studies have practical advantages. These advantages include providing the capacity to answer more than one research question at a time and potentially reducing timelines and costs through a single study set-up. Insights may be gleaned earlier through existing data sources, informing feasibility, generating new research questions, and providing information on the pathway to diagnosis, disease characteristics or treatment patterns. By decreasing the burden of data collection, sites are then able to spend efforts contributing new data that otherwise wouldn’t be available. In addition, clinicians without robust research infrastructures are able to participate in studies, resulting in more representative populations beyond those seen at research-savvy centers. As existing clinical data alone are often not sufficient to fully establish the value of a treatment intervention, the ability of enriched studies to supplement existing data with patient-reported information provides broader perspectives for greater impact.
The benefits of enriched real-world studies are shown in Figure 2.
Design Considerations
The enriched study design is driven by practical considerations, including the availability and accessibility of data elements of interest in existing data sources. The first step is to evaluate what data elements are essential to meet study objectives. The second step is then to assess whether those variables are available in existing data and how complete and accessible they are likely to be. These steps are critical to ensuring that the study’s objectives will be met, and should be done using robust feasibility processes ideally within a subset of the existing EMR or claims data. Treatments, comorbidities and significant healthcare utilization data, such as visits to specialists or hospitalizations, are often reliably collected in existing data systems. However, information such as PROs, QoL, sensor-based data (physical activity, vitals, and adherence), reasons for medication changes and biomarkers are often not available in existing data and are best collected directly from the patient or provider. Similarly, widely accepted clinical measurements that are used in research, like a six-minute walk test or a systematic physician overall assessment of disease activity, may not be consistently available unless collected prospectively.
It is also important to understand that since existing data will have been collected for clinical or administrative purposes, these data will not reflect the consistent use of terminology and data entry practices as would be seen when following a clinical research protocol. Differences in EMR data entry practices are likely to be seen at the clinician, practice and regional or national levels, and even within physicians over the course of a single day. These differences may result in biases (systematic error) and should be addressed in both study design and analysis.
When is an Enriched Study Appropriate?
An enriched study might be appropriate if the following conditions are fulfilled3:
- Some, but not all, critical data elements for addressing the study objectives are routinely found in structured fields within EMR data or insurance claims.
- Study outcomes require or benefit from insurance claims, for example, involving healthcare resource utilization, drug utilization or adherence to treatment (pharmacy dispensing vs. prescription.)
- Study outcomes involve added insight from direct to physician or patient surveys on topics such as reasons for switching or discontinuing treatment, healthcare resource utilization details beyond the reported claims, or patient-reported outcomes and other clinical outcome assessments not available from EMRs.
- A retrospective cohort developed from existing data could serve as a historical comparator to a prospective cohort to meet study goals. This might be particularly appropriate in the setting of a formulary change or breakthrough therapy where no appropriate contemporaneous cohort could be feasibly or ethically created.
- Continuity of care, the ability to follow a patient through both inand out-patient settings, is important to capturing study endpoints for most studies.
- In settings where burden of data collection is a high barrier to study participation, and providers would be more likely to enroll if the majority of data collection could be automated rather than performed by staff.
- In situations where long-term outcomes can be gleaned from existing data, to reduce bias introduced through loss to follow-up.
- EMR/claims data are available for research purposes in the target geographies (such as the United States, European Union or Japan) and are accepted by regulatory agencies.
- Study conduct is occurring in countries where regulations allow identification and contact of patients from the EMR to enable linkage of primary and secondary data sources.
Data Quality Considerations
Having determined that an enriched study is appropriate, the next step is to consider and manage data quality. It is important when using existing data to examine quality and potential for missing data across all required data elements. When designing an enriched study, the following considerations can help identify when primary data collection is required and how to take steps to mitigate risks of missing or incomplete data from existing sources3,4:
- Are data elements recorded in structured fields, as opposed to uploaded via a form (e.g., pathology information) or provided in a text field? Are formats between practices resolvable (e.g. units measured by labs)? Forms may be stored as PDFs which can make it difficult to extract data of interest.
- Is information likely to be recorded accurately and consistently between providers and healthcare systems?
- If a data element will be collected from multiple sources (e.g., claims and EMR or EMR and patient report), which source is considered the Gold Standard? If data is collected from both sources to reduce the potential for missing data, plans for adjudicating discordance between sources should be stated prior to study analyses.
- Feasibility assessments and/or a pilot study may be required to ensure that most, if not all, required data elements are available at the agreed level of quality and completeness. While these activities can be expensive and extend timelines, they can mitigate risks to delivering evidence to meet the study objectives, avoid the need for costly protocol and data collection amendments, and ultimately contribute to the value of these studies.
Attention to adjudication of outcomes, calculation of time windows and compliance with real-world safety reporting implications within good pharmacovigilance practice (GVP) rules are important components of enriched studies. Limitations of existing data sources apply to enriched studies, including variability at the healthcare system and practice level between geographies, entities and EMR systems. Careful evaluation of data elements and qualification of partners can inform decisions on managing missing data and collection of essential data elements.
Case study
Evaluation of usual care practice patterns and referral patterns and how they affect outcomes in management of patients with type 2 diabetes.
Research approach: To address this research question, a longitudinal non-interventional cohort study was designed identifying and following patients through the EMR, supplementing existing data with targeted primary data collection from the site, provider and patient.
Rationale for selecting enriched study:
- Availability of longitudinal data on patient demographics, comorbid conditions, lab values, prescription medication use, and healthcare utilization from the EMR
- allows for efficient and cost-effective data collection
- quick patient recruitment through the EMR
- Certain data are not available within EMR (e.g., site and provider information, frequency of patient measured blood glucose monitoring, and reasons for treatment change)
- these data are best suited for primary data collection directly from the patient and physician
- limited primary data collection reduces site burden and potentially increases participation and representation of the patient population
The cohort of US type 2 diabetes patients was identified using the EMR data with follow-up lasting approximately four years from the time of first patient enrolled. No study visits were mandated; rather, study data was extracted from the EMR via automated function according to what actually occurs in the patient’s practice setting. Data supplemental to the EMR was gathered from the patient, provider and site. Patient reported data was captured via survey completion at baseline and six month intervals via a web-based study portal or third party telephone administrator. Providers captured patient data supplementing the EMR data as dictated by the outcome of each standard of care patient visit. Provider and site level characteristics were captured via survey completion at baseline and 12-month intervals through a web-based study portal.
Operational Findings: The feasibility phase of this study achieved a strong understanding around what data could be reliably collected in the EMR and what data required primary data collection. For example, it was possible to accurately assess the number of doctor’s visits, patient comorbidities and prescription medications through the EMR. On the other hand, while theoretically available, lab values were not always reliably collected in the EMR. In some instances, they were collected but not integrated into the EMR so that the physician could see the imaging of the lab test results as a PDF but the actual lab test values were not stored in the EMR. In another case, the test results were recorded in a notes field that is not accessible to researchers. Prescriptions were often available in the EMR; however, data about whether or not the prescription was actually filled was not. When adherence and refill information is required, linkage to pharmacy claims data is critical.
Understanding availability, accessibility and completeness of data collection in the EMR is critical in enriched studies to ensure required data elements are collected both efficiently and robustly.
Conclusions
Innovative approaches, such as enriched studies that combine primary with secondary data collection, are an emerging option for outcomes research. Enriched studies leverage existing data with de novo data collection to generate meaningful evidence in real-world settings while maximizing efficiency and value.
References
- U.S. Food and Drug Administration, Guidance for Industry, Patient-Reported Outcome Measures: Use in Medical Product Development to Support Labeling Claims, Fed Regist. 2009;74(35):65132-133. Available at www.fda.gov/downloads/Drugs/ GuidanceComplianceRegulatoryInformation/Guidances/UCM193282.pdf. Last accessed August 2013.
- U.S. Food and Drug Administration. Clincial Outcome Assessment (COA): Glossary of Terms. www.fda.gov/Drugs/DevelopmentApprovalProcess/ DrugDevelopmentToolsQualificationProgram/ucm370262.htm#performance. Last updated April 30, 2015. Accessed November 5, 2015.
- Mack CD, McNeill AM, Parmenter L, Davis K, Dreyer NA. Hybrid prospective studies: Combining existing and new data sources to achieve research goals. Pharmacoepidemiology and Drug Safety, Sept 2015; 24(S1): 544.
- Mack, CD, Su Z, Mendelsohn AB, Dreyer NA. Prevention, examination and treatment of missing data in nonexperimental pharmacoepidemiologic studies. Chinese Journal of Pharmacoepidemiology 2015; 24(1): 14-22
Christina Mack is Director, Epidemiology, Real-World & Late Phase Research, Quintiles. Dr. Mack is a pharmacoepidemiologist with global biopharmaceutical experience in applied epidemiologic research, methods development and observational study project management. She has experience with design and analysis of numerous large epidemiologic studies in various real-world data sources and is the lead scientist for the Quintiles COMPASS program, a large distributed data network.
Louise Parmenter, PhD is Global Head of Operations, Epidemiology & Outcomes Research, Real-World & Late Phase Research. Dr Parmenter is a specialist in realworld and late phase research with 23 years of related global operational and strategic experience. She is currently responsible for a team of epidemiologists and outcomes researchers that focuses on safety, comparative effectiveness, design and implementation of Risk Evaluation and Mitigation Strategies (REMS), outcomes research and methods development.
Emma Brinkley is a Sr. Epidemiology Research Associate at Quintiles, responsible for supporting the Scientific Affairs team on real-world and late phase research studies, including study coordination, protocol development, statistical analyses, and analytic report writing. Her work focuses on leveraging existing data for use in enriched studies and pragmatic trails, specifically through the COMPASS Research Network.
Nancy Dreyer, MPH, PhD, is Global Chief of Scientific Affairs for Quintiles’ RealWorld and Late Phase Research division, where she leads an international team in the design, conduct, and interpretation of “real-world” health research, including effectiveness, safety, quality improvement activities and sports injuries. She is a senior editor of “Registries for Evaluating Patient Outcomes: A User’s Guide,” now in its third edition and also leads the GRACE Initiative for developing Good Research Practices for Observational Studies of Comparative Effectiveness.