Introduction
This second article on genotoxic / mutagenic impurities (GTIs) will focus on toxicological aspects and discuss in more detail some of the themes mentioned in the first part of the series [1]. The current [2,3] and evolving guidance [4] is based on a number of key toxicological principles:
- Biological determination, using an appropriate testing protocol, of the presence or absence of DNA-reactivity in a compound that is actually (or potentially) present as an impurity in a drug substance or drug product. A compound that tested positive would be considered to be a GTI.
- Prediction of DNA-reactivity based on the chemical structural alerts of the impurity (denoting electrophilic centers in the molecule), and/or appropriate reference compounds and/or in-silico systems. An impurity possessing a structural alert is often called a potentially genotoxic impurity (PGI) until further data (from biological or in-silico testing) confirm or deny its DNA-reactivity.
- Control of GTIs and/or PGIs using:
- Generic limits for chronic exposure such as the TTC (Threshold of Toxicological Concern [2-3]) when an impurity is shown to be DNA-reactive (GTI) or a worst-case assumption is made regarding a PGI (i.e. that it could be a GTI)
- Compound-specific limits (chronic exposure)
»» Based on linear extrapolation of carcinogenicity potency data
»» Use of standard ICH Q3A [5] qualification thresholds if an in-vivo biological threshold for genotoxic activity can be demonstrated
»» Potentially higher limits based on exposure from other sources, particularly foodstuffs.
- Modification of limits in situations where exposure is less than chronic (LTL – less than lifetime exposure).
Biological Evaluation of DNA-Reactivity
A recent review [6] provides a critical assessment of the current genotoxicity testing paradigm. The Ames bacterial mutagenicity assay, developed in the mid-1970s, has stood the test of time and has gained a strong consensus as the assay of choice for prediction of mutagenicity and carcinogenicity. It has no serious shortcomings for most chemical classes of test substances, and is a highly robust and reliable standalone assay. On the other hand, the most widely used in-vitro assays for mammalian chromosomal damage, for example, the Mouse Lymphoma Assay (MLA), have encountered serious challenges and several reviews have demonstrated that unlike the Ames assay, these in-vitro mammalian genotoxicity assays have poor correlation with rodent carcinogenicity [7,8]. Positive responses in chromosomal-damage assays can occur through a variety of processes including direct DNA damage, delayed or inhibited repair, interference with repair-processing enzymes e.g. topoisomerase, or via artifacts relating to cytotoxicity, apoptosis or culture conditions leading to extreme values for pH and/or osmolality. Mechanisms explaining in-vitro positives in these types of assays are poorly understood at the present time and the value of continuing with clastogenicity-based assays has been seriously questioned. In fact the quite common finding of positive mammalian clastogenicity but negative Ames mutagenicity (often accompanied by negative in-vivo genotoxicity data) has been labelled as a “false positive”, especially when the weight of evidence indicates that the in-vitro positive results arise under conditions not achievable in-vivo e.g. in terms of test material concentration [9] or through indirect mechanisms unlikely to occur at lower clinical concentrations in man.
In order to assess the robustness of the MLA, a dataset of 370 compounds was utilized, comprising a wide variety of chemical structures [10], for which both Ames and MLA results are available (Table 1). There was a false-positive rate (in respect of the non-concordance of MLA vs. Ames) of 44.6% when the positive MLA result was identified using “traditional” criteria (two-fold increase in mutant frequency) and 28.6% when evaluated by proposed “new” interpretation criteria. Thus, in this dataset, the MLA produces an unacceptably high proportion of false-positive results even when conventional mitigating factors are taken into consideration [11]. Worryingly, many of the MLA false positives relate to compounds devoid of conventional structural alerts, some of which are shown in Table 2.
Overall, it can be concluded that data obtained using mammalian cell assays should not be taken into consideration in relation to determining the DNA-reactivity of actual or potential pharmaceutical impurities. This approach is consistent with the current guidance in the EMA Q&A supplement [12] (i.e. if the Ames assay is negative, there is no need for further testing) and with the development of in-silico mutagenicity prediction systems based solely on Ames-test results (see below). On the other hand, as highlighted in the earlier article [1], the EU guideline [2] contains a measure of ambiguity on this issue and does not completely rule out the consideration of mammalian-cell data for Ames-negative impurities.
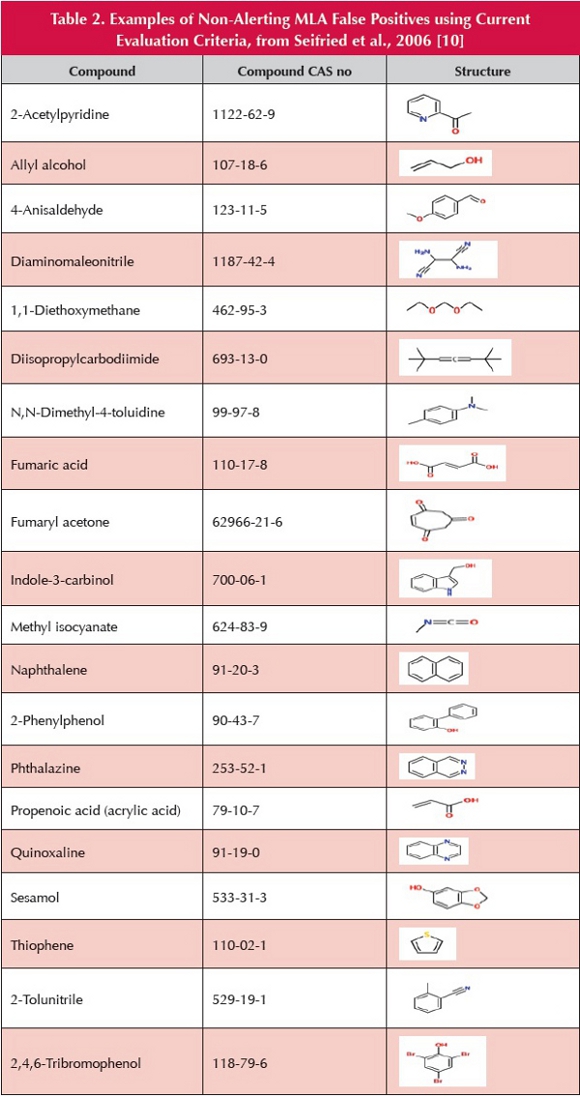
Prediction of DNA-Reactivity
In conventional terms, a compound is considered to be potentially DNA-reactive, i.e. a PGI, if it contains an electrophilic structural feature (e.g., an Ashby-Tennant structural alert for mutagenicity). Tabulations of alerting structural moieties are available in numerous publications [13,14], although it is often difficult or impossible to ascertain the origin and basis of the alerts shown, i.e. does the alert apply to all or just a few compounds with that structural feature?; is the alert associated with either mutagenicity/clastogenicity/carcinogenicity? Recently, structural alerts have been assessed with specific relevance to mutagenic impurities. Kazius et al. [15] evaluated a “training” dataset of 4,337 molecular structures with corresponding Ames-test data, with approximately 55% being assigned as mutagens and the remainder as non-mutagens. Structural alerts were identified using a mixture of data mining and expert knowledge, and the main alerts were identified as aromatic nitros, aromatic amines, strained ring systems (e.g. epoxides, aziridines), nitroso compounds (particularly N-nitrosos), heteroatom-bonded heteroatoms (e.g. N-hydroxy compounds), azo compounds, aliphatic halides and polycyclic aromatic ring systems. Overall, 29 structural alerts were identified along with various detoxifying fragments and an accuracy of 82% was claimed for the mutagenicity status of the compounds in the training dataset. Interestingly, there were some notable exceptions in the Kazius structural alerts compared to those normally presented [13]:
- Saturated alkyl aldehydes: only formaldehyde tests positive, all others being Ames-negative with occasional in-vitro clastogenic responses [16].
- Carbamates: only vinyl carbamate is clearly Ames-positive (based on metabolically mediated epoxide formation), all others appear to be Ames-negative (although occasional high-concentration positives have been reported for ethyl carbamate, and there is one report of a positive response in only TA 1535 with ethyl N,Ndimethylcarbamate). Some carbamates, e.g. propoxur/Baygon (but not methyl or ethyl carbamates), may be MLA-positive [10]. Ethyl and vinyl carbamates are genotoxic carcinogens, the proximate carcinogen being vinyl carbamate epoxide [17]. On the other hand, methyl carbamate is a non-genotoxic carcinogen with a rat NOAEL of 100 mg/kg/day (negative in the mouse) [18].
- Michael acceptors (electron-withdrawing group with adjacent α,β-double bond): Apart from some α,β-unsaturated aldehydes, virtually all Michael acceptors are Ames-negative. Waegemaekers and Bensink [19] evaluated 27 acrylic acid esters in the Ames assay and reported that all gave negative results, whereas alkyl acrylates tend to be MLA-positive [10]. (See also mesityl oxide discussion.)
- N-Oxides: Conventionally, N-oxides of aromatic heterocyclic bases are considered (probably erroneously) to be structurally alerting whereas those of aliphatic or alicyclic tertiary amines are not. N-Oxides of picoline, pyridine and quinoline [20] and levofloxacin [21] are reported to be Ames-negative. Many tertiary amine drug substances (such as trimethoprim and sorafenib) are metabolized to N-oxides, and this kind of transformation is normally associated with detoxification [22]. In some cases the parent aromatic amine may be mutagenic, as is the case with adenine - possibly involving DNA intercalation [22].
A straightforward application of such structural alerts to typical pharmaceutical impurities will give rise to a high proportion of false-positive predictions (and a few false-negatives) owing mainly to a mismatch between structural features present in public domain datasets and the presence of structural elements that diminish or eliminate the effect of the alerting moiety (detoxifying fragments).
At the molecular level the reasons for this complexity are thought to relate mainly to issues of the “chemical space” in the vicinity of the alerting moiety, which could hinder DNA interaction with the reactive center, impede cell entry (in the presence of bulky substituents), alter the hydrophilic-lipophilic balance, impair metabolic activation, etc. QSAR (quantitative structure-activity relationships) systems that correlate physicochemical parameters with mutagenic potential have been partially successful in terms of their predictive ability particularly for homologues within a series of structurally similar congeners [6].
The use of in-silico systems for predicting mutagenic activity is of enormous interest across many areas of toxicology, not just pharmaceuticals, owing largely to the potential to reduce or eliminate biological testing. Some believe that the use of QSAR computational toxicology in relation to pharmaceuticals has many advantages [6]:
- Methodology is cheaper and faster than biological testing and can provide information on mechanism and mode of action
- In-cerebro QSAR assessment using conventional structural alerts is considered obsolete by some, but by no means all toxicologists, since:
- Many different pharmaceutical-specific structural alerts exist
- It does not account for detoxifying fragments, whereas a computer-based system can evaluate all structural alerts and detoxifying motifs
- Machine learning/statistical models, which are available only to in-silico systems, can usefully complement structural alert-based approaches.
- Ability to use two or more systems which is expected to improve the accuracy of prediction.
In-silico expert systems fall into three main categories: rule-based systems such as Toxtree [23] and Derek [24], statistical/machine-learning models such as TOPKAT [25], MultiCASE [26] and CAESAR [27], and hybrid systems that combine knowledge-based and statistical-based approaches [28].
The overall accuracy of mutagenicity prediction (taking into account both positive and negative predictions) is normally reported as 70-75% using various commercial and open-source systems. There are many publications on the use of in-silico mutagenicity prediction systems; an estimate of at least 100 such papers was cited recently [28], and many more have been published subsequently involving training/benchmark datasets of up to nearly 10,000 compounds. The general picture that emerges using either single or combined in-silico models is that systems using public-domain datasets (as is normally the case) perform adequately to predict the genotoxic potential of compounds in the same or similar “applicability domains” (ADs), but are deficient when challenged with pharmaceutical impurities, which typically have higher molecular weights, higher lipophilicity and more complex structures [29,30].
Hillebrecht et al. [31] used an Ames dataset of 9,681 compounds (public and proprietary) to evaluate the performance of four proprietary in-silico systems. Accuracy and specificity were broadly similar when evaluating public data, but sensitivity was significantly reduced for proprietary data. The expert systems generally showed higher sensitivity and lower specificity when compared to QSAR-based tools, which displayed the opposite behavior. Performance differences between the public and proprietary data are likely to relate to the proportion of Ames +/- compounds in the datasets and to “chemical space” issues. Dobo et al. [29] reported that much higher prediction rates (99%) were attained using one or more commercial/in-house in-silico systems combined with expert human interpretation. Interestingly, using more than one in-silico system failed to improve the accuracy of prediction. However, this apparently impressive performance appeared, in general, to be greatly aided by the fact that the impurity structures evaluated fell into the “company-specific chemical space.”
The CAESAR (Computer Assisted Evaluation of industrial chemical Substances According to Regulations) system [27, 32] is an open-source software tool that employs a dataset of 4,337 compounds for training purposes. The overall accuracy of predicting both positive and negative Ames results is claimed to be 85%. The system has a two-stage design and comprises a machine learning algorithm from the Support Vector Machines (SVM) collection, to build an initial model with the best statistical accuracy, then an ad-hoc expert system based on known structural alerts tailored to refine the initial predictions. The performance of the CAESAR system has been evaluated using a series of structurally alerting alkyl chloride compounds, most of which are reported as testing Ames-negative. The compounds involved, the CAESAR predictions and literature data are shown in Table 3.
The key conclusions are:
- Data on 2/8 compounds (both correctly recorded as Amesnegative) are present in the underlying training dataset.
- 7/8 compounds were predicted to be suspect mutagens, even the two compounds reported as Ames-negative in the original dataset.
Thus, it seems that for this particular group of compounds, CAESAR [32] performed extremely poorly, and it appears that the various C-Cl structural alerts were given undue weight in the predictions. Another facet of this small group of compounds is that Ames-testing using normal procedures gives negative results, but when tested in the desiccator assay (often employed for volatile substances), 4/8 compounds were positive. The positive results on three of these compounds employ only one strain of Salmonella and are not accompanied by control data, and so may not be particularly reliable.
Sucralose (Figure 1) possesses a considerably more complex structure than the compounds in the training dataset, having three separate C-Cl structurally alerting motifs, but it also contains five hydroxyl groups which could be considered to act as detoxifying elements since the compound is non-metabolized, tests negative in the Ames and other genotoxicity assays, and is also non-carcinogenic [38]. Many in-silico systems would, most likely, predict sucralose and other chlorodeoxy sugars to be DNA-reactive since a chlorine attached to an aliphatic carbon is potentially susceptible to nucleophilic displacement, in contrast to an aromatic chloro compound such as chlorobenzene. A more holistic approach based on the metabolic stability of particular chloro sugars, rather than identifying alerting molecular fragments, is more appropriate for this class of compounds (and possibly others). An ADI (acceptable daily intake) of 0-15 mg/kg/day has been determined by all of the major food agencies for sucralose [39], suggesting that an intake up to 1.5 g/day would be safe for a 100 kg adult (106 times greater than the default TTC).
An additional evaluation using CAESAR has been made for mesityl oxide (4-methylpent-3-en-2-one, Figure 2). Mesityl oxide, possesses a conventional α,β-unsaturated ketone structural alert, and is often identified as a PGI in drug substances that have been crystallized from acetone, as it is a potential impurity in this solvent.
Although mesityl oxide is reported to be Ames-negative [40], some reviewers have requested a repeat Ames assay “conducted to regulatory acceptable standards” [16].
In conclusion, it is clear that there are numerous uncertainties in respect of using structural alerts and in-silico systems for evaluating PGIs. An additional potential issue concerns the need to ensure that there is a “level playing field” in terms of DNA reactivity assessed by in-silico systems (whose underlying datasets are based to a large extent on public domain data) and by direct citation of a specific publication. Whereas, the latter might well suffer “push-back” during regulatory review (as for mesityl oxide), with an attendant demand for a “gold-standard” Ames assay, in-silico predictions are gaining increasing regulatory acceptance without the need for confirmatory biological studies.
Threshold of Toxicological Concern (TTC)
During the development of regulatory guidance on GTIs, a key factor concerned the limits to apply to a GTI and/or a PGI a GTI, or a PGI for which no clear prediction concerning the absence of mutagenic activity could be made. As explained in the previous paper [1], the critical issue for such impurities is DNA-reactivity as relates to potential carcinogenicity. Hence an apparently logical solution was to create an approach based on carcinogenicity potency data for a wide variety of compounds with structural alerts representative of those present in pharmaceutical impurities. The Threshold of Toxicological Concern (TTC), whose origins lie in the food rather than the pharmaceutical sector, was adopted as a “ready made” solution. Kroes et al. [41] identified a group of genotoxic and nongenotoxic carcinogens (700+) from the Carcinogenic Potency Database (CPDB) [42] and employed model-free linear extrapolation of their TD50 values; i.e. a measure of carcinogenic potency which, in simple terms, is the dose in mg/kg/day associated with the occurrence of treatment-related tumors in 50% of the test animals in a conventional rodent lifetime bioassay.
In order to predict the doses with an increased cancer risk of ≥ 1 in 106 in humans over a lifetime (assumed to be 70 years), the TD50 values were divided by 500,000, yielding a value for the virtually safe dose (VSD) for each compound (in mg/kg/day). Assuming a human bodyweight of 60 kg, the doses (which range from 0.15-150 μg/ day) associated with a carcinogenic risk of 1 x 106 were tabulated for different structural classes (Table 4). The largest numbers of compounds were either aromatic amines (162) or N-nitrosos (105), and the most potent were aflatoxins-like compounds, azoxy compounds and N-nitroso compounds (≥ 45% of these three compound groups had a carcinogenic risk of ≥ 1 in 106 at a dose of 0.15 μg/day). Kroes et al. [41] included these three structural types in the “cohort of concern”, and these were omitted from the scope of the TTC.
Kroes et al. [41] then recommended that a global TTC of 0.15 μg/day could be determined for compounds containing a structural alert for carcinogenicity (but not mutagenicity). It was recommended that compounds within the cohort of concern should be evaluated on a compound-specific basis. Due to the enhanced risk / benefit ratio applicable to pharmaceuticals, an increased risk of 1 in 105 was considered relevant when drawing up guidance on genotoxic impurities, thus producing a TTC of 1.5μg/day. The EU guideline [2] indicates that: “It should be recognized in this context that the methods on which the original TTC value is based, are generally considered very conservative since they involved a linear extrapolation from the dose giving a 50% tumor incidence (TD50) to a 1 in 106 incidence, using TD50 data from the most sensitive species and most sensitive sites (i.e. several “worstcase” assumptions).” Note also that the EU guideline [2] and the draft FDA guidance [3] refer to a structural alert relating to genotoxicity as a prerequisite for applying the TTC limit.
Numerous shortcomings in the derivation of the TTC, including those mentioned above concerning the use of data from the most sensitive tissue, gender, species in rodent bioassays, have been identified [43]. Overall, the current derivation of the TTC in relation to genotoxic impurities can be considered as flawed and non-transparent [43]; it contains multiple layers of over-conservatism and bias such as the use of atypical TD50 values (lowest statistically significant oral TD50 as used by Cheeseman et al. [44]), employing data only on compounds judged to be carcinogenic [43,44], and excluding structurally alerting noncarcinogens (a significant number of which are present in the core CPDB database [42, 43]), employing only one highly conservative extrapolation technique (inappropriate for non-genotoxic carcinogens and structurally alerting/genotoxic non-carcinogens), and ignoring the fact that nongenotoxic carcinogens are normally considered to be thresholded with respect to dose-response relationships [46,47]. In addition, the final step of the Kroes procedure (in relation to genotoxic impurities) relies on just 16 compounds in the highest risk group (Table 4) and, unfortunately, the identity of these key compounds cannot be ascertained with certainty in order to evaluate whether they truly represent genotoxic carcinogens (and not non-genotoxic carcinogens or non-carcinogens). A reevaluation of the TTC based only on structurally alerting compounds in the CPDB with extrapolation techniques tailored to the genotoxicity/carcinogenicity status of the compounds should be eminently possible.
Control of Ames-positive GTIs and PGIs without Ames Data
A variety of strategies are available for managing Ames-positive GTIs. If none of the approaches mentioned below is applicable, then the default TTC limit is considered appropriate.
Oncology Products
In the context of mitigating genotoxic risk in oncology APIs and drug products, ICH S9 is highly relevant [48]. The guideline indicates that conventional limits for GTIs are not appropriate for pharmaceuticals intended to treat patients with advanced cancer, and, with justification, higher limits can be applied (i.e. ICH Q3A [5]). This approach is based on the premise of a shortened lifespan for cancer patients, the likelihood that the API is genotoxic / carcinogenic or cytotoxic in its own right and the fact that the concomitant medications used in cancer therapy are also likely to be genotoxic / carcinogenic or cytotoxic. Against this background, the additional risk arising from the presence of a GTI in the drug substance is considered negligible. In contrast, Callis et al. [49] propose a slightly higher limit of 30 μg/day in oncology products, administered on a daily basis, over a 2-year period. It is interesting, that other disease states where a similar shortened lifetime (< 2-years) can be anticipated, e.g. many inborn errors of metabolism (Phenylketonuria, Gaucher’s disease, Zellweger syndrome, etc), do not appear to have been explicitly excluded (in a similar manner to ICH S9 [48]) from existing genotoxic impurity guidances [2,3].
Purity of Test Material for Ames Assays
Impurities in test materials can be a confounding factor in Amespositive findings. Levels as low of 0.15% of highly potent genotoxins such as 2-aminoanthracene or sodium azide [50] can produce positive results. Hence a test-material purity of 99%+ is recommended to provide absolute assurance that the finding is truly applicable to the impurity in question. Assessment of individual results from each of the five bacterial strains used in the Ames test can be useful in delineating any ambiguity. Thus if other compounds in this particular alerting class typically elicit a positive effect in TA 98, but the GTI in question elicits a positive effect in TA 1538 and an alkylating agent used as a precursor in the synthesis of the GTI would also likely elicit a positive effect in the same strain (TA 1538), then this strongly suggests that the positive findings may be attributable to an impurity in the test material (e.g. a reagent used in the synthesis of the impurity). In which case, purification of the test material followed by a repeat of the Ames test is warranted.
A well documented example is for impurities present in 3-nitro-9- fluorenone. The original sample gave a strongly positive result in TA 98 strain of Ames test. However, after column purification the purified sample was 6-times less potent. Two extremely potent impurities (2,7-dinitro-9- fluorenone and 2-acetamido-3-nitro-9-fluorenone) were isolated and both tested positive in the Ames assay [50].
Thresholded GTIs
If a threshold can be identified for the specific GTI, then the NOAEL (no observed adverse effect level) or LOAEL (lowest observed adverse effect level) is divided by a suitable safety factor to establish a PDE (permitted daily exposure) as outlined in ICH Q3C [51]. A recent example of this approach applies to EMS (ethyl methanesulfonate – present in the ethanolcontaminated reagent methanesulfonic acid), which was identified as an impurity in Viracept (nelfinavir mesylate) leading to the product’s temporary withdrawal in Europe during 2007 [52]. The marketing authorization holder [52] showed that EMS is thresholded in terms of determination of a NOAEL of 25 mg/kg/day in subchronic study in transgenic mice. A PDE of 104μg/day was derived based on ICH Q3C criteria [51], i.e. around 70-fold greater than the default TTC [2]. Müller et al. [52] indicated that ‘although the findings cannot currently be extrapolated to other genotoxins, it has become obvious that the generally applied assumption of linear dose relationships for mutagenicity (and by default carcinogenicity) for one of the most typical alkylating agents is not based on fact.’ Müller et al. [53] concluded that ‘the TTC approach (based on linear extrapolation) should not be seen as being set in stone even for DNA-damaging genotoxins.’ A combination of compound-specific toxicokinetic data together with the human-equivalent threshold dose for DNA alkylation imply a limit in the region of 10-100mg/day [54], although a limit of no more than the one mentioned above (ca. 100μg/day) seems to be favored by regulators [55].
If no threshold can be assumed or derived, linearity is often assumed (despite EMS findings) and the applicable risk assessment techniques depend on available data. In respect of deciding on a suitable testing paradigm for establishing a threshold for an Ames-positive impurity, clear guidance is currently lacking. It is to be hoped that an evidence-based series of follow-on tests can be devised that enables “staged” limits to be applied depending on the outcome of individual/multiple tests.
GTIs with Carcinogenicity Data Available: Use of Carcinogenicity Data to Set Limits
As mentioned above, the conventional approach in regulatory toxicology is to distinguish between genotoxic (non-thresholded) and nongenotoxic carcinogens (thresholded). For the latter, a compound-specific risk assessment can be made by applying a variety of assessment factors to the NOAEL determined in a chronic toxicity/carcinogenicity study. Limits for five Class 2 solvents were derived in this way [56]. Unfortunately, extrapolation of carcinogenicity potency data, strictly applicable only to genotoxic carcinogens, has also been used in the derivation of the TTC and to obtain limits for non-genotoxic carcinogens. As shown in Table 5, much lower limits are produced by linear extrapolation of carcinogenicity data compared to those obtained by conventional NOAEL/assessment factor techniques employed in ICH Q3C [51]. The principal potency metrics employed in the risk assessment of genotoxic carcinogens are the TD50, BMD and T25 [57].
TD50
The full definition of the TD50 is as follows: “For any particular sex, strain, species and set of experimental conditions, the TD50 is the dose rate (in mg/kg body weight per day) that, if administered chronically for a standard period (the “standard lifespan” of the species), will halve the mortality corrected estimate of the probability of remaining tumorless throughout that period”. Values for specific compounds can be accessed via the CPDB. Linear extrapolation at a risk level of 1 in 105 of the TD50 value for the particular compound can be used to determine a suitable limit [55, 58]. To determine a PDE (permitted daily exposure) or VSD (virtually safe dose), the TD50 is divided by 50,000 (linear extrapolation to risk of 1 in 105) and multiplied by 50 (standard patient body weight specified in ICH Q3C [51]). Thus, the PDE will have the same value as the TD50, but in units of μg/day rather than mg/kg/day. It should be remembered that a PDE/VSD determined in this manner is highly conservative, being based on lifetime exposure. It is generally recognized that linear extrapolation of the most appropriate TD50 value is the simplest and possibly the most robust approach to determining a PDE/VSD for a genotoxic impurity for which carcinogenicity data are available.
T25
The T25 potency index [57] is defined as “the chronic dose rate in mg/ kg body weight per day, which will give 25% of the animals tumors at a specific tissue site, after correction for spontaneous incidence, within the standard lifetime of that species”. The T25 was proposed as a simplified method not requiring sophisticated statistical methods and computer software, and to be specifically used in regulatory settings when a ranking according to carcinogenic potency is deemed necessary for classification of carcinogens. The T25 is presently used within the European Union for various regulatory purposes.
BMD
The BMD [57] is the benchmark dose. The BMD10 (the dose that gives rise to a 10% tumor increase) or BMDL10 (the lower 95% confidence limit of the BMD10) is used as the point of departure (POD) for risk assessment in a particular animal carcinogenicity study. The BMD values are determined by statistical modelling of the tumor incidence data (Figure 3). Using linear extrapolation from the POD, a cancer slope factor (as used in the IRIS database by the Environmental Protection Agency [59]), or a cancer potency factor [60], can be determined and limits derived accordingly (normally based on tumor risk of 1 in 100,000). Limits based on cancer potency factors derived by OEHHA (Office of Environmental Health Hazard Assessment) are reported as NSRLs (no significant risk levels) [61]. A comparison of NSRLs for several typical genotoxic carcinogens with limits obtained by linear extrapolation of TD50 data is shown in Table 6.
No Carcinogenicity Data Available
In those cases where carcinogenicity data are not available, the TTC is applied by default unless the applicant can demonstrate that higher levels are justifiable based on duration of exposure or based on preexisting exposure, e.g. via foodstuffs. One example is crotonaldehyde for which, even in non-drinking, non-smoking consumers, exposure via food is estimated to be around 150 μg/day [62]. A further interesting example is N-nitrosamines for which there are independent confirmatory reports that dietary/endogenous human exposure is
typically 100 μg/day [63,64]. The specific nitrosamines involved include two that are noncarcinogenic (N-nitrosoproline and N-nitrosohydroxyproline) and several that are highly potent (N-nitrosodimethylamine, N-nitrosopyrrolidine and N-nitrosopiperidine) with TD50 values ranging from 0.1-1.3 mg/kg/ day. N-Nitrososarcosine appears to be less potent but it is still classified as a likely human carcinogen [17]. These data suggest a potential conflict with the notion of all N-nitrosamines being part of the “cohort of concern” [2,3].
Staged TTC or LTL (less than lifetime) Concept
Although this topic was discussed previously [1], it is helpful to consider the toxicological basis of permitting higher levels of GTIs when less-than-lifetime exposure is involved. A key publication is by Bos et al. [65] which is referenced in the draft FDA guideline [3] but not in the EU guideline [2]. According to Bos et al. [65], the starting point for any evaluation is the VSD, which is based on lifetime exposure. The TTC is just a generic VSD, rather than a compound-specific value, and so the staged TTC/LTL concepts can be viewed as derivatives of the more general approach based on VSD. Therefore, it is completely logical to be able to apply an LTL adjustment to a compound-specific VSD as well as to a TTC limit.
Conclusions
Although the main elements of regulatory guidance on GTIs have been in place for several years, there is still considerable scientific uncertainty on many key toxicological issues. For example, structural alerts are not clearly defined or agreed, the role of data from mammaliancell assays remains unclear, the current TTC appears somewhat biased and certainly overly conservative and the methodology for its derivation is inconsistent with prior art established for non-genotoxic solvents in ICH Q3C. Furthermore, the introduction of in-silico techniques into the regulatory arena brings with it a number of critical issues on the appropriate number of independent systems, data integrity and the role of expert interpretation. Various risk assessment techniques are available for non-genotoxic and genotoxic carcinogens and it is currently unclear which are likely to be more or less acceptable. Overall, facile solutions are not possible for many issues and it is to be hoped that eventual ICH guidance will acknowledge such problems and permit a range of approaches provided that they are scientifically justified.
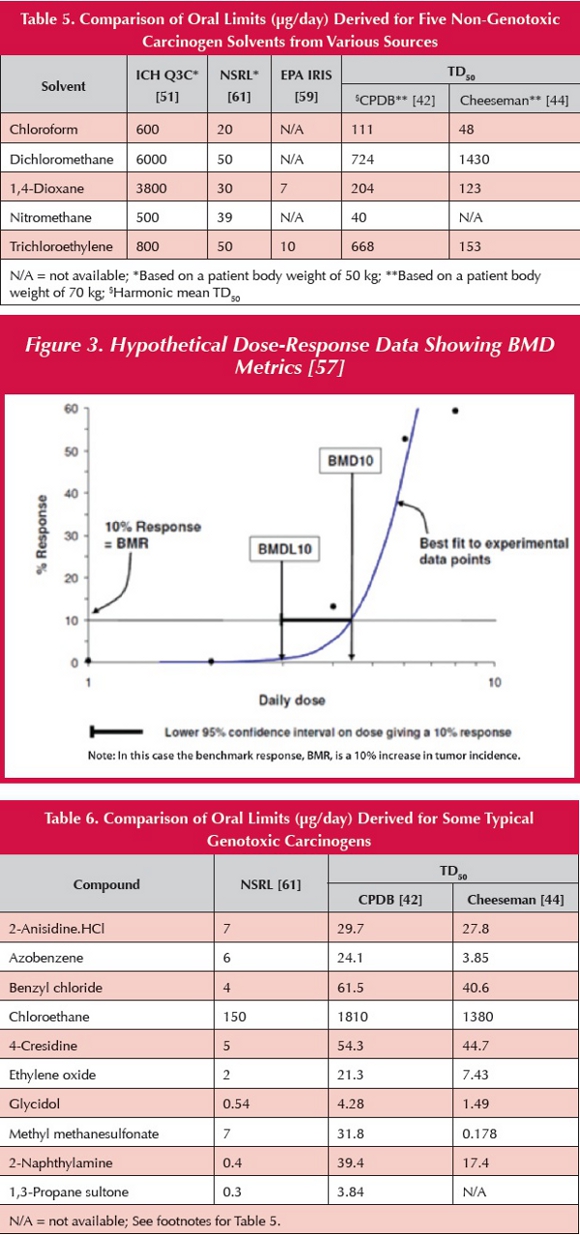
Acknowledgements
The authors would like to acknowledge the constructive and helpful comments made to the manuscript during drafting by Jim Harvey and Amanda Giddings (GSK) and Andy Teasdale (AstraZeneca).
References
1. D.P.Elder, D.J.Snodin. Genotoxic Impurities Part 1: General Overview. Pharmaceutical Outsourcing, 13(3), 2012, 44-59.
2. Committee for Medicinal Products for Human Use (CHMP), Guideline on the limits for genotoxic impurities, 01 January 2007.
3. Guidance for Industry, Genotoxic and carcinogenic impurities in drug substances and products: Recommended approaches, U.S. Department of Health and Human Services, Food and Drug Administration, Center for Drug Evaluation and Research (CDER), December 2008.
4. P. Kasper. Controlling of genotoxic impurities in pharmaceuticals: international harmonisation of regulatory requirements. Toxicology Letters. 205S. 2011. S05-3. 5. ICH Q3C, Impurities in new drug substances, in International Conference on Harmonization Tripartite Guideline. June 2008, Revision 2. http://www.fda.gov/downloads/ RegulatoryInformation/Guidances/ucm127984.pdf. Accessed on 30th August 2012.
6. B.Mahadevan, R.D.Synder, M.D.Waters, R.D.Benz, R.A.Kemper, R.R.Tice, A.M.Richard. Genetic toxicology in the 21st century: Refl ections and future directions. Environ. Mol. Mutagen., 52(5), 2011, 339-354.
7. D.Kirkland, M., Aardema, L., Henderson, L., Müller, Evaluation of the ability of a battery of three in vitro tests to discriminate rodent carcinogens and non-carcinogens. I. Sensitivity, specificity, and relative predictivity. Mutat. Res., 584, 2005, 1–256.
8. E.J.Matthews, N.L. Kruhlak M.C. Cimino, D.R. Benz, J.F. Contrera. An analysis of genetic toxicity, reproductive and developmental toxicity, and carcinogenicity data: I. Identifi cation of carcinogens using surrogate endpoints Reg. Toxicol. Pharmacol., 44, 2006, 83–96
9. J.M. Parry E. Parry P. Phrakonkham R. Corvi. Analysis of published data for top concentration considerations in mammalian cell genotoxicity testing. Mutagenesis, 25(6), 2010, 531-538.
10. H.E.Seifried, R.M.Siefried, J.J.Clarke, T.B.Junghans, R.H.C.San. A compilation of two decades of mutagenicity test results with the Ames Salmonella typhimurium and L5178Y mouse lymphoma cell mutation assays. Chem. Res. Toxicol., 19, 2006, 627-644.
11. ICH S2(R1), Guidance on Genotoxicity Testing and Data Interpretation for Pharmaceuticals Intended for Clinical Use, Current step 4 version 1, 09th November 2011: http://www.ema.europa.eu/docs/en_GB/ document_library/Scientifi c_guideline/2011/12/WC500119604.pdf. Accessed on 01st August 2012.
12. European Medicines Agency, Questions and Answers on the ‘Guideline on the limits for genotoxic impurities’, EMA/CHMP/SWP/431994/2007 revision 3, 23rd September 2010. Accessed on 31st July 2012.
13. L. Mueller, R.J. Mauthe, C.M. Riley, M.M. Andino, D. De Antonis, C. Beels, J. DeGeorge, A.K.M. De Knaep, D Ellison, J.A. Fagerland, R. Frank, B. Fritschel, S. Galloway, E. Harpur, C.D.N. Humfrey, A.S. Jacks, N. Jagota, J. MacKinnon, G. Mohan, D.K. Ness et al., A rationale for testing, and controlling specified impurities in pharmaceuticals that posses the potential for genotoxicity. Reg. Toxicol. Pharmicol., 44, 2006, 198-211.
14. K.L. Dobo, N. Greene, M.O. Cyr, S. Caron, W.W. Ku, The application of structure based assessment to support safety and chemistry diligence to manage genotoxic impurities in active pharmaceutical ingredients during drug development. Reg.Toxicol. Pharmacol., 44, 2006, 282-293.
15. J.Kazius, R.McGuire, R.Bursi. Derivation and validation of toxicophores for mutagenicity prediction, J.Med. Chem., 48, 2005, 312-320.
16. D.J. Snodin, Genotoxic Impurities: From Structural Alerts to Qualification, Org. Proc. Res. Dev., 14, 2010, 960-976.
17. http://toxnet.nlm.nih.gov/. Accessed on 23rd August 2012.
18. http://ec.europa.eu/food/fs/sc/scf/reports/scf_reports_39.pdf. Accessed on 23rd August 2012.
19. T.H. Waegemaekers, M.P. Bensink. Non-mutagenicity of 27 aliphatic acrylate esters in the Salmonella-microsome test. Mutat. Res., 137(2-3), 1984, 95-102.
20. C.E. Voogd, J.J, van der Stel, J.J. Jacobs. The mutagenic action of quindoxin, carbadox, olaquindox and some other N-oxides on bacteria and yeast. Mutat Res., 78(3), 1980, 233-42.
21. Q. Zhu, T. Li, J. Li, M. Guo, W. Wang, X. Zhang. In silico and in vitro genotoxicity evaluation of levofloxacin N-oxide, an impurity in levofloxacin. Toxicol. Mech. Methods, 22(3), 2012, 225-230.
22. J.W. Gorrod, C. Ioannides, S.P. Lam, S. Neville., Mutagenicity testing of 9-N-substituted adenines and their N-oxidation products. Environ Health Perspect., 101(3), 1993, 21-26.
23. Toxtree, Toxic Hazard Estimation by decision tree approach. http://toxtree.sourceforge.net. Accessed on 01st August 2012.
24. DEREK, Deductive Estimation of Risk from Existing Knowledge, https://www.lhasalimited.org/ index.php/derek_nexus. Accessed 0n 01st August 2012.
25. TOPKAT, www.chem.ac.ru/Chemistry/Soft/TOPKAT.en.html. Accessed 0n 01st August 2012.
26. MultiCASE, http://www.multicase.com. Accessed 0n 01st August 2012.
27. CAESAR, Computer Assisted Evaluation of industrial chemical Substances According to Regulations. http://vega.marionegri.it/wordpress/resources/qsar-in-silico-tools. Accessed on 01st August 2012.
28. R.Serafimova, M.F.Gatnick, A.Worth. Review of QSAR models and software tools for predicting genotoxicity and carcinogenicity. JRC Scientific Report EUR 24427 EN-2010: http://publications.jrc. ec.europa.eu/repository/handle/111111111/14330. Accessed on 08th August 2012.
29. K.L. Dobo , N. Greene, et al. In silico methods combined with expert knowledge rule out mutagenic potential of pharmaceutical impurities: an industry survey. Regul Toxicol Pharmacol., 62(3), 2012, 449-55.
30. R.T. Naven, S. Louise-May, N. Greene. The computational prediction of genotoxicity. Expert Opin. Drug Metab. Toxicol., 6(7), 2010, 797-807.
31. Hillebrecht, W. Muster, A. Brigo, M. Kansy, T. Weiser, T. Singer. Comparative evaluation of in silico systems for Ames test mutagenicity prediction: scope and limitations. Chem. Res. Toxicol., 24(60), 2011, 843-54.
32. T.Ferrari, G.Gini. An open source multistep model to predict mutagenicity from statistical analysis and relevant structural alerts. Chem. Cent. J., 4(1), 2010, 1-8. http://www.ncbi.nlm.nih.gov/pmc/ articles/PMC2913329/ Accessed on 08th August 2012.
33. E.Eder, T.Neudecker, D.Lutz, D.Henschler. Correlation of Alkylating and mutagenic activities of allyl and allylic compounds: Standard alkylating test vs. kinetic investigation. Chem. Biol. Interact., 38, 1982, 303-315.
34. V.F. Simmon, K. Kauhanen, R.G. Tardiff. Mutagenic activity of chemicals identified in drinking water. In Progress in genetic toxicology: Proceedings of the Second International Conference on Environmental Mutagens (Vol 2), D. Scott, B. Bridges, F. Sobel (Eds), 1977, pp. 249-258.
35. http://toxnet.nlm.nih.gov. Accessed on 01st August 2012.
36. V.F.Simmon. Application of Salmonella/Microsome Assay. in: Short-Term Tests for Chemical Carcinogens; H.F.Stich, R.H.C.San (Eds.), Springer-Verlag, New York, 1981, pp.120-126.
37. IUCLID Dataset for ethyl monochloroacetate : http://www.epa.gov/chemrtk/pubs/summaries/ ethyl/c13406rr.pdf. Accessed on 07th August 2012.
38. D. Brusick, V.L. Grotz, R. Slesinski, C.L. Kruger, A.W. Hayes. The absence of genotoxicity of sucralose. Food Chem. Toxicol., 48(11), 2010, 3067-3072.
39. V.L. Grotz, I.C. Munro. An overview of the safety of sucralose. Regul. Toxicol. Pharmacol., 55(1), 2009, 1-5.
40. Inert Reassment – mesityl oxide: http://www.epa.gov/opprd001/inerts/ mesityl.pdf. Accessed on 07th August 2012.
41. R. Kroes, A.G. Renwick, M.Cheeseman, J. Kleiner, I.Mangelsdorf, A.Piersma, B.Schilter, J.Schlatter, F. van Schothorst, J.G. Vos, G.Würtzen. European branch of the International Life Sciences Institute. Structure-based thresholds of toxicological concern (TTC): guidance for application to substances present at low levels in the diet. Food Technol. Toxicol. Lett., 42, 2004, 65-83.
42. Berkeley Carcinogenic Potency Database (http://potency.berkel.edu/ pdfs/herp.pdf). Accessed on 01st August 2012.
43. D.J.Snodin, S.D. McCrossen. Guidelines and pharmacopeial standards for pharmaceutical impurities: Overview and critical assessment. Reg. Tox. Pharmacol., 63, 2012, 298-312.
44. M.A. Cheeseman, E.J. Machuga, A.B. Bailey. A tiered approach to threshold of regulation. Food Chem. Toxicol., 37(4), 1999, 387-412.
45. Scientific Opinion on Exploring options for providing advice about possible human health risks based on the concept of Threshold of Toxicological Concern (TTC). EFSA Journal 10(7, 2012,) 2750. http:// www.efsa.europa.eu/de/efsajournal/doc/2750.pdf. Accessed on 07th August 2012.
46. G.M. Williams , J. Whysner. Epigenetic carcinogens: evaluation and risk assessment. Exp. Toxicol. Pathol., 48(2-3), 1996, 189-95.
47. European Scientific Committees. Risk assessment methodologies and approaches for genotoxic and carcinogenic substances’, 2009. http:// ec.europa.eu/health/ph_risk/committees/04_scher/docs/scher_o_113. pdf. Accessed 07th August 2012.
48. ICH S9: Nonclinical Evaluation for Anticancer Pharmaceuticals, in International Conference on Harmonization Tripartite Guideline. EMEA/CHMP/ICH/646107/2008.Assessed on 01st August 2012.
49. C.M. Callis, J.P. Bercu, K.M. DeVries, L.K. Dow, D. K. Robbins, D.L. Varie. Risk Assessment of genotoxic impurities in marketed compounds administered over a short-term duration: Applications to oncology products and implications for impurity control limits. Org. Proc. Res. Dev., 14, 2010, 986-992.
50. Z.L. Zin, X.B. Xu, H.P. Hsu, J.P. Nachtman, E.T. Wei. Potent Mutagenic Impurities in a Commercial Sample of 3-Nitro-9-Fluorenone. Cancer Letts., 15, 1982, 209-214.
51. ICH Q3C. Guideline for Residual Solvents, in International Conference on Harmonization Tripartite Guideline. EMEA/CPMP/ ICH/28395/2009.Accessed on 30th August 2012.
52. L. Müller, T. Singer. EMS in Viracept —The course of events in 2007 and 2008 from the non-clinical safety point of view. Tox. Letters, 190, 2009, 243-247.
53. L. Müller, E. Gocke, T. Lavé, T. Pfister. Ethyl methanesulfonate toxicity in Viracept —A comprehensive human risk assessment based on threshold data for genotoxicity. Tox. Letters, 190, 2009, 317-329.
54. D.J. Snodin, A.C. Cartwright, B.R. Matthews. Production statement for mesilate-salt drug substances is no longer justified. Pharmeuropa Readers’ Tribune, April 2012, 1-10.
55. P. Kasper. Global regulatory challenges for controlling genotoxic impurities – Regulatory perspective from the EU. AAPS Annual Meeting. Los Angeles 2009: http://mediaserver.aaps.org/ meetings/09AM/Slides/11.12.09_Thu/409%20AB/1330/Peter%20 Kasper.pdf. Accessed on 29th August 2012.
56. http://www.fda.gov/downloads/Drugs/ GuidanceComplianceRegulatoryInformation/Guidances/UCM073401. pdf. Accessed on 23rd August 2012.
57. A Harmonised Approach for Risk Assessment of Substances which are both Genotoxic and Carcinogenic. http://www.efsa.europa.eu/en/ efsajournal/doc/282.pdf. Accessed on 23rd August 2012
58. J.F.Contrera. Improved in silico prediction of carcinogenic potency (TD50) and risk specic dose (RSD) adjusted threshold of toxicological concern (TTC) for genotoxic chemicals and pharmaceutical impurities. Regul. Toxicol. Pharmacol., 59, 2011, 133-141.
59. Guidelines for carcinogen risk assessment. US Environmental Protection Agency. http://www.epa.gov/cancerguidelines/. Accessed on 23rd August 2012.
60. OEHHA Cancer potency factors. http://www.oehha.ca.gov/air/hot_ spots/tsd052909.html. Accessed 29th August 2012.
61. Proposition 65 No Significant Risk Levels for Carcinogens. http:// oehha.ca.gov/prop65/pdf/2012StatusReportJune.pdf (Accessed 29th August 2012)
62. Concise International Chemical Assessment Document 74: 2-butenal. http://www.inchem.org/documents/cicads/cicads/cicad74.pdf. Accessed 29th August 2012.
63. A.R. Tricker. N-nitroso compounds and man: sources of exposure, endogenous formation and occurrence in body fluids. Eur. J. Cancer Prev., 6(3), 1997, 226-268.
64. P. Jakszyn, S. Bingham, et al. Endogenous versus exogenous exposure to N-nitroso compounds and gastric cancer risk in the European Prospective Investigation into Cancer and Nutrition (EPIC-EURGAST) study. Carcinogenesis, 27(7), 2006, 1497-1501.
65. P.M.J.Bos, B.Baars, T.M.Marcel, M.T.M. van Raaij. Risk Assessment of Peak Exposure to Genotoxic Carcinogens: A Pragmatic Approach. Toxicol. Lett., 151, 2004, 43-50.
David Snodin studied Chemistry at Imperial College, London, moving to Bristol University to undertake research for a Ph.D. in Organic Chemistry. He later obtained an M.Sc. in Toxicology at the University of Surrey. He has been involved in regulatory toxicology for over 30 years, initially in industry, then at the UK Medicines and Healthcare products Regulatory Agency (Expert Preclinical Assessor and UK representative on the CHMP Safety Working Party). He was recently employed for 8 years by PAREXEL International Drug Development Consulting as Vice President – Nonclinical. In February 2010 he formed his own consulting business – Xiphora Biopharma Consulting, specializing in nonclinical consulting generally with a particular interest in the Quality-Safety interface (particularly involving safety assessment of impurities and excipients) and environmental risk assessment. His recent publications are focused on the safety evaluation of genotoxic and nongenotoxic pharmacopoeial and other impurities.
David Elder studied chemistry at Newcastle upon Tyne, before moving to Edinburgh University to undertake research for a Ph.D. in Crystallography. Dr. Elder has 34-years of experience in the pharmaceutical industry at a variety of different companies (Sterling, Syntex and GSK). For the last 19 years, he has been employed by GSK. He is currently a director in the externalization group (Scinovo) in GSK R&D. Dr Elder is a member of the British Pharmacapoeia (Expert Advisory Group PCY: Pharmacy), a member of the Analytical Division Council (Royal Society of Chemistry, UK) and a council member of the Joint Pharmaceutical Analysis Group, UK. He is a member of the PhRMA and EfPIA sub-groups on genotoxic impurities and was part of the PQRI group that assessed the control strategies for alkyl mesilates. His recent publications are focused the chemistry/analytical evaluation of genotoxic impurities. He has authored three chapters in the recently published Genotoxic Impurities: Strategies for Identification and Control (Ed. Andrew Teasdale, Wiley, 2010.
This article was printed in the September/October 2012 issue of Pharmaceutical Outsourcing, Volume 13, Issue 5. Copyright rests with the publisher. For more information about Pharmaceutical Outsourcing and to read similar articles, visit www.pharmoutsourcing.com and subscribe for free.